Waymo, a veteran of autonomous driving, said:
Our AI drivers were able to avoid 75% of crashes and 93% of serious injuries, all higher than ideal human driver models of 62.5% and 84%.
Wait, did you find the problem? What is Waymo's basis for such a precise quantitative description of autonomous driving safety? The point of Waymo's latest paper isn't to show off how safe autonomous driving is, at least not at all. AEB (active braking) has become standard, and smart cars have been shouting for many years. However, each company has different standards. How much speed and what kind of obstacles can AEB or emergency avoidance take effect to be considered a qualified product?
Waymo's real goal is to try to develop a set of norms to define and evaluate whether a self-driving system is safe. In the future, any company that says that autonomous driving is safer than human driving needs to be supported by a scientific computing system, rather than simply comparing road tests and accidents under different conditions.
What is the benchmark for evaluation: Modeling human reaction times?
One of Waymo's contributions is that they have developed a new architectural model to measure and model driver reaction time in real-world road conditions. In fact, simply put, it is to compare the reaction time of an autonomous driving system with the average reaction time of human drivers in emergency situations. This architecture is not only suitable for autonomous driving, but also for other areas of traffic safety. Specifically, the model is based on two core ideas:
First, in order to avoid a collision, drivers often choose to brake or turn the steering wheel. They made this move mainly because the current traffic situation was different from what they had thought, and the driver was surprised. That is, the reaction time depends on the driver's anticipation of the current traffic situation. When the surprise and surprise start will directly determine the length of the reaction time. Second, the reaction time depends on the dynamically changing traffic environment. There is no one-size-fits-all set time that can be applied to all different scenarios.
For example, if the car in front of you brakes suddenly, you can react quickly; on the other hand, other things being equal, if the car in front slows down slowly, your reaction time will be extended accordingly. It should be noted that the reaction time here refers specifically to the driver's psychological process of deciding whether to brake or turn and does not include subsequent evasive actions (ie, turning the steering wheel or stepping on the brakes). The diagram below can better explain their model architecture. The whole process can be summed up as the belief updating process. In the upper part of the picture, after seeing the traffic lights, the driver naturally thinks that the car in front needs to brake to slow down, but the fact is that the car in front did brake and slow down. So the driver's prediction was correct and matched the actual result, and in this case, the driver did not appear a "surprise".
In the lower part of the picture, the driver thought that the car in front was going to continue moving forward, but the fact was that the car in front suddenly braked, which was inconsistent with his psychological expectations, and the cognition was updated iteratively.
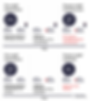
The following diagram further explains the entire process of cognitive transformation.

The emergence of this model architecture is mainly to solve the two major limitations of the previous reaction time modeling:
The reaction time is too dependent on the surrounding environment;
How to define "stimulus" clearly.
Waymo hopes to measure the reaction time of humans from seeing obstacles to pressing the brakes in a real road environment, facing various intricate driving environments. Under the traditional method, the analysis of reaction time is generally based on specific controllable experiments, and it is not possible to clearly define when the "stimulus" in common traffic accidents is triggered. With such a more rigorous reaction time benchmark model, the performance of the autonomous driving system can be evaluated.
The human driver as the reference model
In order to judge how well Waymo's own AI drivers are performing, in addition to the reaction time model mentioned above, a standard and reference are needed. NIEON came into being. It is a reference behavior model, an ideal human driver, named after the initials of each word in the series Non-Impaired Eyes ON the conflict. This means that NIEON drivers have no intellectual, hearing, or visual impairments. They stay focused during the entire driving process, and will not be distracted or drowsy. Comparing Waymo's AI driver with the NIEON model, the results are:
In the collision avoidance effect (collision avoidance effect), and being involved in 16 traffic accidents at the same time, Waymo's automatic driving system was able to avoid 12 collisions, that is, the avoidance probability reached 75%.
Note: This specifically refers to the collision avoidance response, excluding the conflict avoidance effect, which means that you change your trajectory and speed to avoid an accident/reduce the severity of an accident, or regain control after you lose control the vehicle.
In contrast, the ideal NIEON model avoids 10 collisions with a probability of avoidance of 62.5%.

At the same time, Waymo's self-driving system can reduce 93% of serious injuries caused by collisions; the NIEON model can only reduce 84%. That's why Waymo came to the conclusion that their self-driving AI drivers are safer than older human drivers. The paper says that a behavioral reference model similar to NIEON can be used as a benchmark to judge the quality and safety of a set of ADS autonomous driving. As for whether the test results are reliable, Waymo officials also talked about 4 limitations in the paper.
First, the dataset they are currently using involves collisions that are primarily caused by humans. Of course, it's important to consider how an automated driving system can handle these known, human-induced crashes correctly, and to test the system's ability to avoid similar behavior.
Second, the study only modeled reconstructions based on police-reported crashes, and the number of crashes recorded in official documents may differ from reality. Third, the current study is only based on a single NIEON model operation to judge the quality of Waymo's self-driving system. Fourth, the performance of the entire autonomous driving system is tested in a simulated environment and under different conditions. If it is a challenge for some specific scenarios, it is not suitable for this method in the strict sense.
Without showing off the data, what's the point of Waymo's two articles?
Let's start with one of the most common questions: why is it difficult for autonomous driving to land? On the surface, the regulations are imperfect, and the division of rights and responsibilities for autonomous vehicles is not clear. But we might as well consider it from the perspective of the legislature. Why is the division of responsibility for autonomous driving unclear in today's L2-L3 stage?
Quite simply, current self-driving systems are not perfect enough to be "foolproof" and require human drivers to be ready to take over. The timing and conditions of this takeover have never been clear. There is no qualitative and quantitative standard to define under what circumstances humans need to take over the system, and naturally, it is impossible to clearly divide rights and responsibilities legally.
Therefore, the source of imperfect regulations is not the lag of legislation, but the entire autonomous driving industry, which has never provided the legislature with technical standards that can work at the legal level. Even the L0-L5 grading of the industry-wide SAE is based on a perceptual description of the degree of human intervention, rather than a scientifically rigorous quantitative description.
To clear the obstacles to the implementation of autonomous driving at the regulatory level, it is necessary to give exact and rigorous definitions in terms of system reliability, road complexity, system capability boundaries, human intervention conditions, and critical points of system failure. Waymo's two papers are aimed at the dimension of the reliability of the autonomous driving system, and quantitatively calculate the reliability of the autonomous driving system based on the reaction time. It is said that autonomous driving is more reliable than human driving. How reliable is it?
Tesla’s previous words are based on the comparison of the number of accidents of non-autonomous vehicles by the US traffic control department and the number of Tesla accidents. But the problem with this is that the road conditions, vehicle conditions, and accident types across the United States are too complex, far beyond the scene coverage of the automatic driving database. Some accidents that humans cannot avoid are not necessarily avoided by FSD, and conclusions can only be drawn by repeating the experiment under the same conditions. But this is obviously unrealistic. This is also the confusion of Tesla's commercial propaganda.
And Waymo's model and method, I dare not say that it will become the industry standard, but at least it is a good start for the definition of autonomous driving safety. Of course, Waymo’s two papers also have a meaning, that is, once again, it has been popularized to the public that autonomous driving is not equal to 0 accidents. Even high-level autonomous driving systems such as L4 and L5 still have the risk of failure. The significance of autonomous driving is that the risk of system error and failure is lower than that of humans, which can greatly promote the efficiency of social and economic operations. This point has now been rigorously proved and has the basis for legislation.
Read more here